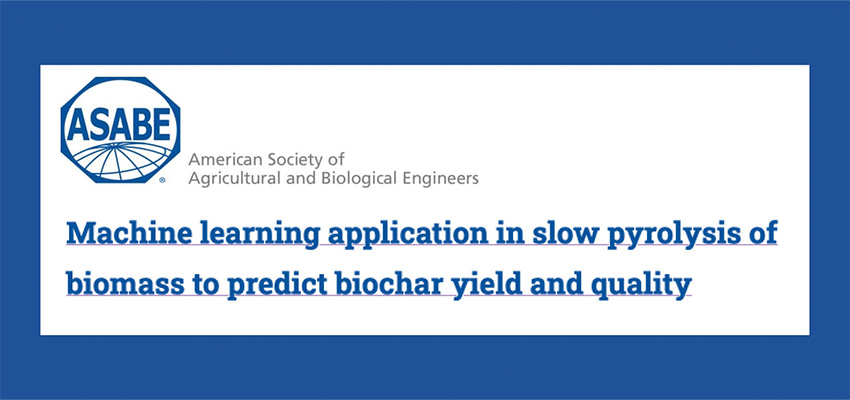
American Society of Agricultural and Biological Engineers (ASABE) publications page
Abstract
High-quality biochar production is crucial since it has numerous applications in agriculture, energy, and climate change. Optimizing slow pyrolysis to obtain high-quality biochar via an experimental approach is complex, expensive, and time-consuming. On the other hand, machine learning can be used to understand and optimize the slow pyrolysis process by leveraging published experimental data. In this study, the yield and quality (elemental carbon percentage) of biochar were predicted from experimental data using random forest (RF) machine learning algorithm. The biochar yield showed a strong negative correlation with pyrolysis temperature (p < 0.01, r = -0.612), while the elemental carbon content of biochar showed a significant positive correlation (p < 0.01) with both temperature (r = 0.699) and feedstock elemental carbon content (r = 0.570). The RF model showed that the pyrolysis temperature accounted for 57% and 50% of the variation in biochar yield and quality, respectively. A partial dependence plot analysis was also performed to understand the influence of each feature on the predicted outcome. According to the results, the important factors arranged in ascending order of importance for biochar yield were pyrolysis temperature (57%), feedstock ash content (14%), and feedstock elemental carbon content (8%). Future predictions should include a broader range of features and more data points.
More information
MIT D-Lab Biomass Fuels & Cookstoves Research
Contact
Dan Sweeney, MIT D-Lab Research Scientist
Priyabrata Pradhan, Fulbright Postdoctoral Researcher