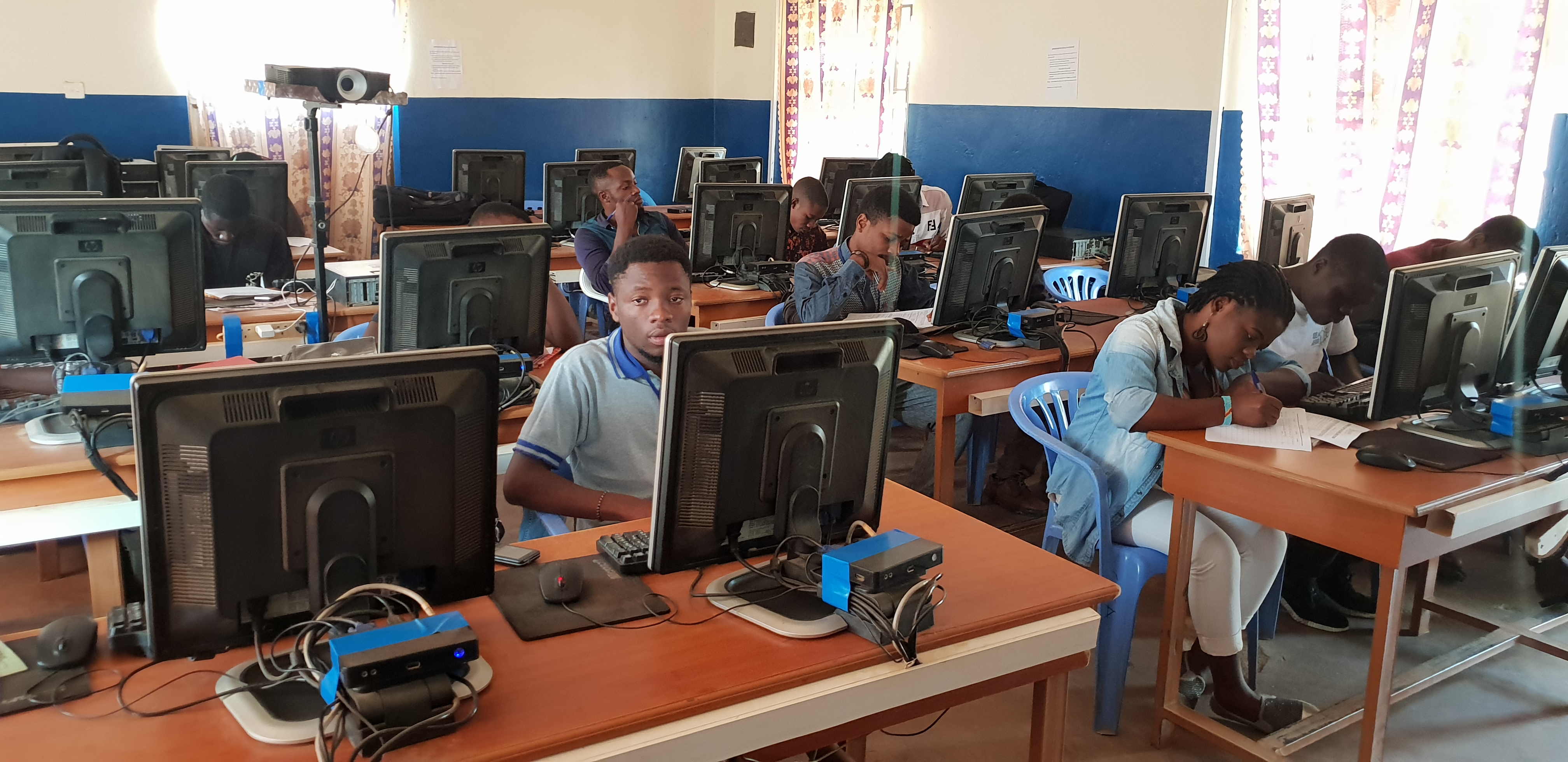
As a D-Lab Monitoring and Evaluation (M&E) Fellow working for Kyusa this summer, I had the rare opportunity of consulting with an organization that had already taken several steps to establish its monitoring and evaluation system.
Kyusa is a Ugandan non-profit founded by Noeline Kirabo, one of MIT D-Lab’s 2018 Innovation Ecosystem Builder Fellows. Kyusa addresses youth unemployment in Uganda’s slums by empowering young people to turn their passions into sustainable careers. Over the last few years, they have invested more resources in understanding the effects that their programs have had on their participants.
By the time I arrived in Uganda, Kyusa had already administered nearly 150 surveys to participants of its entrepreneurship trainings. But how effective were these surveys? At this stage, it was important to carefully analyze the effectiveness of the instruments that Kyusa has in place before moving any further on our evaluation of the organization’s impact. This got us working on an important interactive exercise: the survey assessment.
After analyzing all 150 responses to Kyusa’s survey, I was able to gain important insights about the merits and quality of each question being asked. This was before I had even administered the survey myself. I did so by calculating the response rates on each question, assessing the types of responses that were being provided, and having an honest conversation with Noeline about whether or not the answers to each question were adding to Kyusa’s knowledge on its own performance. Here is a breakdown of the process we followed.
What is the response rate on each question?
A question’s response rate can be a good indicator of its quality. Comparing a question’s response rate to other questions within the same survey can shed light on how respondents are reacting to that particular question. Low response rates should be taken as red flags. Those questions could be difficult to understand or too sensitive for participants to feel comfortable answering. Alternatively, they could be too time consuming, or the information being asked may not be readily available to the respondent. Another possibility is that the question may be difficult to see and easy to skip over.
Sometimes, the solution may be as simple as adding multiple choice answers to an open-ended question. For instance, Kyusa had included an open-ended question about the respondent’s industry. This question had a relatively low response rate, likely because many respondents did not necessarily think of themselves as being part of a larger industry. Adding categories for industries could both help increase the response rate and narrow down the number of industries specified.
However, the reasons why a question has a low response rate may not always be obvious. If you have any doubt, it is always smart to simply ask your respondents the next time you administer the survey. People’s reactions may surprise you!
Do any questions have a significant number of “wrong” answers?
It is not always easy to spot a “wrong” answer in a survey, but there are a few places to look for clues. First, the answers to some questions may be inconsistent with the answers to others. For example, if a significant number of the people that have never owned a business are reporting that they have employed people, then either the question on business ownership or employment may have an issue. Second, “wrong” answers can look like extremes or outliers. For example, it is unlikely that a person would have more than 15 children to support. The question on the number of children being supported may be misunderstood as the number of beneficiaries supported by the respondent’s NGO. Finally, “wrong” answers tend to be more common in open-ended questions. People may be using different currencies if you haven’t specified a currency on your income question, or they could be reporting the number of objects sold instead of their value on your sales question. It’s important to identify why a question is being answered incorrectly, especially if it is a common occurrence. Rewording a question to be more specific, adding instructions, and setting field rules in digital surveys (like minimum and maximum values) can often help provide more accurate answers.
Is everyone giving the same response to any of your questions?
If 100% of respondents answer the question in the same way, then it should probably either be revisited or removed, even if you are getting an answer that you like. For example, 100% of respondents to Kyusa’s survey said that the training has met their expectations. That’s an answer that makes Kyusa look good, but is it really telling us anything useful? Perhaps replacing that yes/no question with a scale may tell us more about the degree to which expectations were fully met and their level of enthusiasm.
Here’s what this question told us. Is that useful information?
Even when nothing stands out about how people are responding to a question; it may still need to be changed. When assessing the effectiveness of your survey, it is important to reflect on how useful the aggregated and analyzed answer to each question actually is to your work. While you may have thought that an indicator was necessary when your first devised the survey, it may not serve you anymore.
Alternatively, your questions may not serve the indicators the way that you had intended. For example, if you want to know whether the number of people that the entrepreneur employed increased following your training, then asking “How many people have you employed?” may not capture this increase. Instead, asking “How many people are you currently employing?” will probably help you more accurately measure the change following the intervention.
As the Kyusa team and I learned this summer, developing an M&E system is a continuous process. The data collected from your survey is not just data about your performance; it also contains data that can help you further fine tune your M&E system itself. Armed with this data, Kyusa has a new-and-improved survey instrument. Yet, the bulk of the 150 responses to its previous survey will still be included in its M&E dataset, since the new instrument was mostly geared to improving responses through rewording the same questions. The actual meaning of most questions was not changed. With a little extra effort, we were able to both improve Kyusa’s survey and maintain continuity in the organization’s data.
About the Writer
Fatema Alhashemi was a Monitoring and Evaluation fellow at MIT D-lab. She is currently pursuing an MPA in Development Practice and an MA in Quantitative Methods in the Social Sciences at Columbia University. Fatema has conducted program evaluations in Morocco, the Western Sahara, and India, and is currently finalizing two case studies on the outcomes of different public sector reforms in Jordan and Egypt for the Brookings Institution Press.
More information
D-Lab Innovation Ecosystem Builder Fellowship